Prediction Timers
Troubleshoot prediction latency, find bottlenecks and optimize performance
JFrog ML prediction timers help troubleshoot model prediction latency, measure prediction times, find bottlenecks, and optimize performance.
Configure multiple timers with custom names for clear visibility using either a context manager or a decorator.
After building, deploying and sending inference requests to your model, you can view these timers on the Latency breakdown graph.
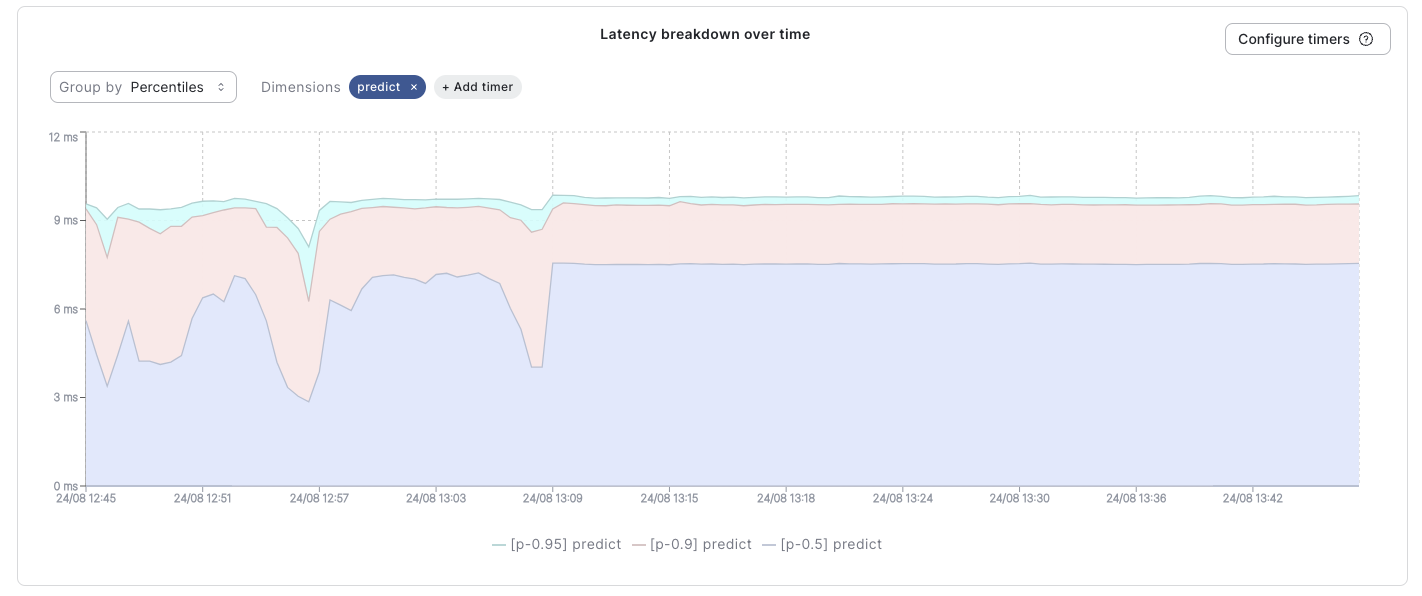
See the JFrog ML prediction timer in the Model Overview
Note: The prediction timer graph may display up to 5 timers including the default
overall
andpredict
timers. This leave room for additional 3 custom timers.
Configure timers via decorators
JFrog ML timers may be configured via a function decorates manager, to easily wrap different methods that are called during the prediction process.
from qwak import qwak_timer
from qwak.model.base import QwakModel
class MyModel(QwakModel):
def build():
pass
@qwak_timer("my_custom_timer_name")
def pre_process(self, df):
# Perform custom processing
return df
def predict(self, df):
self.pre_process(df)
return df
Configure timers via context managers
JFrog ML timers may be configured via a context manager, to easily wrap parts of your code that need measurements.
from qwak import qwak_timer
from qwak.model.base import QwakModel
class MyModel(QwakModel):
def build():
pass
def predict(self, df):
with qwak_timer("my_custom_timer_name"):
# Perform custom processing
df = df + 1
return df
Default timers
Overall timer
Measure the end to end inference time, starting at the moment a request arrived, until the inference output.
Queue timer
Measures the the time it takes requests to leave the input queue before reaching the model.
Understanding Latency Metrics
When analyzing your model runtime latency charts, it's important to distinguish between two key metrics we track: Overall
and Predict
latency.
Overall Latency: This metric provides a comprehensive view of the request lifecycle, measuring the time elapsed from when a request reaches our load balancer to the moment a response is sent back to the user. This includes several infrastructure-level processes, such as:
- Request routing mechanisms
- Authentication and authorization procedures
- Network communication between containers
Predict Latency: In contrast, the "Predict" metric offers a more granular perspective, specifically focusing on the execution time of your Python predict
method.
While the "Overall Latency" encompasses factors largely outside your direct control, several components within it can be influenced by your configuration:
- Webserver Queueing: The duration requests spend waiting in the webserver before processing begins.
- Serialization/Deserialization: The time taken to convert data into and out of formats suitable for transmission and your model (heavily influenced by your chosen adapter and the size of the data).
- Context Switching: The overhead associated with switching between different workers and threads within your deployment.
- Model Loading: The time required to load your model into the memory of your worker processes (particularly significant when the number of workers exceeds available threads).
Optimizing Overall Latency:
You can generally improve these controllable latency factors by adjusting your resource allocation strategy. Key parameters to consider include:
- Instance Type: Selecting an instance type with appropriate CPU, memory, and network capabilities.
- Instance Count: Scaling the number of deployed instances to handle the incoming request volume.
- Workers per Instance: Configuring the number of worker processes allocated to each instance.
Note: Increasing the number of workers does not always result in better performance. It may lead to increased latency due to more frequent model loading operations and overload from excessive context switching.
Conclusion
By understanding the factors affecting latency and implementing strategic resource allocation, you can optimize the performance of your systems and improve overall responsiveness.
Updated about 1 month ago