What is JFrog ML?
Everything ML engineering and data science teams need to build and scale ML in production.
About JFrog ML
JFrog ML simplifies the process of building, deploying, and monitoring machine learning models, bridging the gap between data scientists and engineers as a unified AI solution for every need.
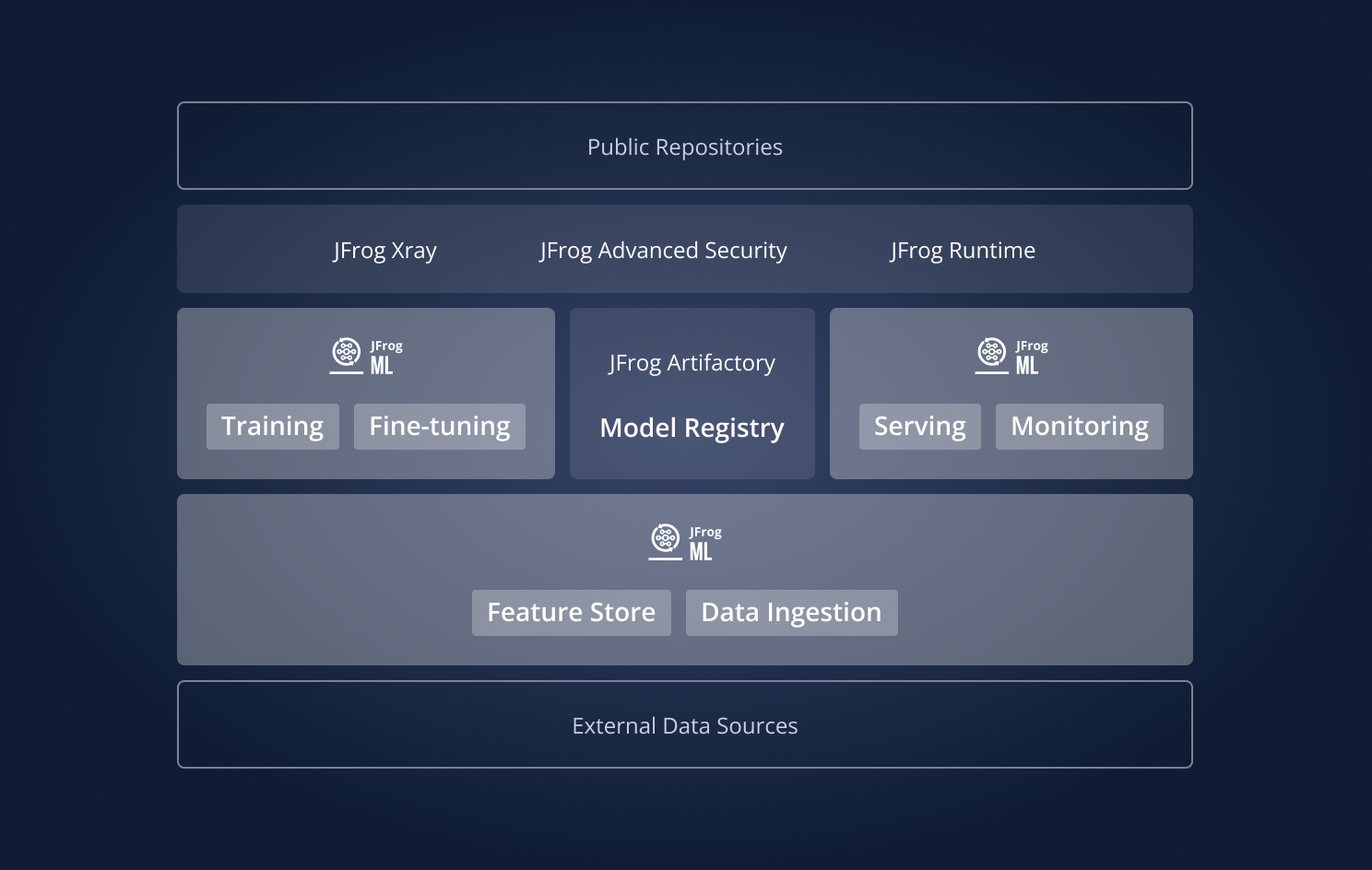
The entire ML model life cycle from research to production
Use JFrog ML to build and manage the entire Machine Learning life cycle. Easily prepare data, build, train and deploy models, monitor, and automate your pipelines, all in one place.
The first ML engineering platform
Take your machine learning models from research to production faster and with more confidence with JFrog ML, including:
- Deploying and iterating on your models faster
- Testing, serializing, and packaging your models using a flexible build mechanism
- Deploying models as REST endpoints, batch transformation jobs or streaming applications
- Gradually deploying and A/B testing your models in production
- Querying model results and visualizing model behavior in production
ML production observability
Gain complete control and visibility of your machine learning production environment with JFrog ML observability features. Easily deploy builds, view performance metrics, manage model features, and analyze and query your inference models.
Automated feature pipelines
Simplify the process of building feature pipelines with JFrog ML Feature Store.
Create batch, streaming and real-time feature transformations with end-to-end pipeline visibility. Seamlessly integrate these pipelines with JFrog ML-based models using the platform's powerful and automatic feature extraction mechanism.
Flexible deployment models
Choose the deployment option that works best for your business needs.
JFrog ML Cloud provides quick and secure deployment, while the JFrog ML Hybrid architecture allows you to secure your data and models in your own cloud environment.
Updated 5 days ago